A Study on the Application of CNN to Mountain Tunnel Face Observations with an Examination of a Face Photography Method
1. Introduction
In mountain tunnels, engineers conduct an operation known as a "face observation," which involves observing and evaluating the state of a given excavation cross-section (face), and then select a support pattern. However, the results of a face observation can vary depending on the experience of the engineer conducting it. This has led to various attempts to apply AI (such as image analysis technology and the like) in face observations in recent years. However, because conditions for applying AI have yet to be established, unclear results reliability and other uncertainties are thought to exist. In view of this, we are verifying the accuracy of face observations conducted using CNN (convolutional neural networks) and examining the conditions required for face photographs used in CNN.
2. CNN-based analysis
First, we trained a model with training data that labeled evaluation points for the adopted support pattern, weathering and alteration, and other items in face photographs. We then examined the percentage of correctness of the model's face observation results and where on the face the AI focused its attention when we inputted new face photos.
The results showed percentages of correctness of around 70 to 80%. However, as is shown in Figure 1, many of the results focused on areas unrelated to the face, such as previously constructed support members and obstacles. Consequently, it is important for engineers to make comprehensive judgments that are based not only on correctness percentages but also on the quality of the photographs used in the training data and the validity of the AI's decision-making process.
3. Photographic conditions for face photographs
Based on the study outlined in the previous section, we examined the photographic conditions that are required for the face photographs used in AI-conducted face observations. Specifically, we placed a resolution chart in front of a face and took about 2,000 face photographs by combining different camera settings (such as the number of pixels and f-number) and photography environments (such as face luminosity, etc.). We then evaluated their resolution performance by reading the resolution chart (5 points for high resolution and 1 point for low resolution). From here, we analyzed the relationship between the evaluation points and resolution by proposing the following equation, which we derived by considering image brightness, amount of noise, and other aspects.
Camera setting Evaluation Score (1) = F-number/(shutter speed × ISO sensitivity)
Camera setting Evaluation Score (2) = Evaluation Point (1) × number of pixels (10,000)
![]() Evaluation Score (1) and resolution |
![]() Evaluation Score (2) and resolution |
4. Conclusion
We are currently conducting actual on-site verifications. We plan to use the results in reviewing photographic conditions and then to add or consider new points that deserve attention when taking photographs.
(Contact: Tunnel Research Team)
Measuring Avalanches from the Air: 3D Avalanche Measurement with Drones
1. The difficulty of measuring avalanches
Avalanche causes serious damage, when it occurs.
It is necessary to understand the whole image of an avalanche, including its volume, in order to consider effective emergency measures, warnings and evacuations for preventing additional damage in the same location.
However, it is not easy.
One reason is that deep snow prevents people from approaching closely to the avalanche's source.
Another is that traces of the avalanche change immediately from moment to moment due to continuous snowfall or thawing.
For solving this problem, it is required to develop the technology that can quickly measure an avalanche site without approaching avalanche directly.
2. Measuring avalanches with drones
So, we: Snow Avalanche and Landslide Research Center, focused on the use of drones, a technology that has made remarkable advances in recent years.
Until now, the airplane has been the main technology used for surveying the ground from the air.
However, using airplanes is expensive and has disadvantages regarding to mobility.
On the other hand, Drones can quickly reach the site of an avalanche immediately after it occurs.
And Drones can calculate the avalanche volume and other characteristics by taking measurements in a short time and at low cost.
Recreating 3D terrain by compositing photos taken in a series, called "structure from motion" or "SfM", is one method of taking 3D measurements by drone.
We had apprehension to use SfM for avalanche.
It was thought not to be able to compose the photograph, because avalanche is pure white when it was photographed by Drone.
3. Taking on the challenge of making 3D avalanche measurements
Figure 1 shows drone flying on actual avalanche site.
Measurement by SfM can be applied by normal small drone.
Figure 2 shows a 3D model that was created from photos taken by the drone using dedicated software.
As can be seen in Figure 2, we succeeded in creating a 3D model of the measured site including its white snow surface without major absences of detail.
In the case of Figure 2 ①, the terrain and trees made it difficult to see the entire area from the ground.
However, with the 3D model, it was possible to grasp the area's entirety from various angles on a desktop.
In the case of Figure ②, we can estimate the avalanche volume by measuring the depth which was created from the 3D model.
If the amount of avalanche can be grasped, it will be possible to determine the scale of emergency measures.
Moreover, if the risk of re-expansion can be grasped from snow cracks, it can be useful for warning and evacuation.
Snow Avalanche and Landslide Research Center will continue to study cases including accuracy verifications.
We are planning to create a document that understands the survey method and summarizes it, so that the findings obtained in this research can be utilized in the actual field.
(Contact: Snow Avalanche and Landslide Research Center)
Research Study on Hydrodynamic Pressure Generated in Irrigation Pipelines during Earthquakes
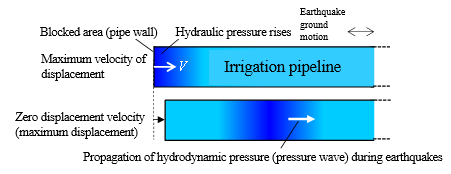
generation in a pipeline during an earthquake
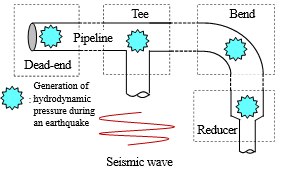
is generated in a pipeline during an earthquake
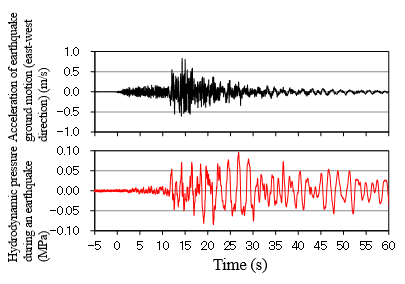
(seismic intensity of 4)
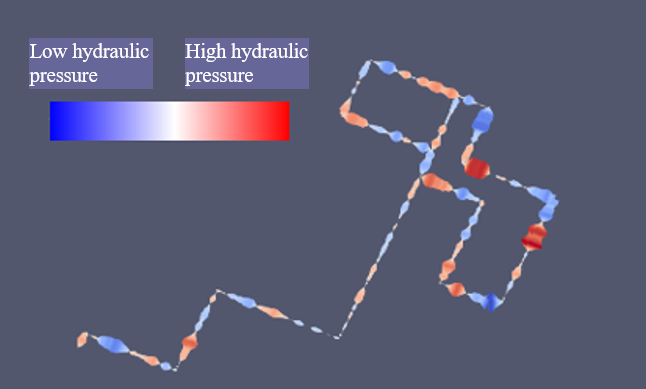
hydrodynamic pressure during an earthquake
(Distribution of hydrodynamic pressure in a pipeline
several seconds after the occurrence of
hydrodynamic pressure during an earthquake)
Irrigation pipelines (hereinafter referred to as "pipelines") through which river water flows from dams and headworks to be distributed to agricultural land form an important social infrastructure that supports food production in Japan. Many issues related to the design, construction, service, and management of pipelines have been overcome by the efforts of our predecessors. However, there are still unsolved issues regarding earthquake countermeasures for pipelines. One of the issues concerns measures against hydrodynamic pressure during earthquakes.
As shown in Figure 1, at blocked areas of a pipeline, the hydrodynamic pressure (hydraulic pressure rise or fall) is generated by the pipe wall that is displaced due to earthquake ground motion and that instantly pushes (or pulls) the water to flow in the pipe. The hydrodynamic pressure also becomes a pressure wave that is propagated in the pipe to fluctuate the hydraulic pressure at any point in the pipeline. This change in hydraulic pressure is the hydrodynamic pressure that occurs during earthquakes. In addition to the blocked areas of the pipeline, hydrodynamic pressure during earthquakes also occurs at bends, tees, and reducers (Figure 2).
Such hydrodynamic pressure during earthquakes has long been considered as one of the causes of damage to pipelines during earthquakes. However, until recent years, there have been few cases of observing hydrodynamic pressure in pipelines in service during earthquakes, and the actual state of hydrodynamic pressure in pipelines during earthquakes could only be presumed.
In the wake of the Great East Japan Earthquake, the Irrigation and Drainage Facilities Research Team began observing the hydrodynamic pressure in a pipeline in service during earthquakes. This observation consists of waiting for an earthquake to happen and constantly obtain acceleration data from the ground as well as hydraulic pressure data inside the pipeline. So far, we have obtained data from more than a dozen earthquakes with a seismic intensity of 2 ? 4.
Figure 3 shows an example of the observation results. It was confirmed that hydraulic pressure is generated during an earthquake due to ground motion.
t was also found that the magnitude of the hydrodynamic pressure during an earthquake does not decrease even after earthquake ground motion has declined, and may be further amplified. We are currently developing a program that uses the characteristic curve method, and are conducting a simulation that reproduces the observed data. Figure 4 shows an example of the simulation results. We have confirmed the situation that hydrodynamic pressure generated in bends during earthquakes causes interference that is amplified during the process of being propagated in the pipeline.
In the future, we will accumulate observation data of hydrodynamic pressure during earthquakes, improve the accuracy of the model, and make full use of numerical simulations to elucidate the destruction process of pipelines due to hydrodynamic pressure during earthquakes. Based on the results, we also aim to develop countermeasure technology to
reduce earthquake damage in pipelines.
(Contact: Irrigation and Drainage Facilities Research Team, CERI)